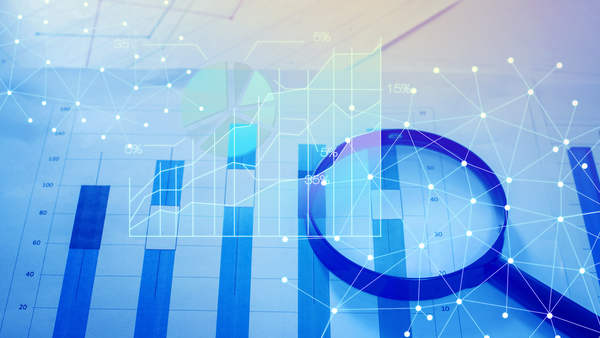
If an anomaly occurs in a KPI but your analysis tools are unable to spot it, did it really happen?
Today almost every business is a data-driven business. Whether it’s the monthly scrap rate at a factory or the conversion rate of website visitors to sales, each business has one or more critical metrics. These key performance indicators (KPIs) are indicators of the success and health of the company. Each data point from a KPI is a snapshot of that particular quantity at that particular time: The factory scrap rate for March, or last Tuesday’s percentage of website visitors who actually bought something.
Once You’ve Got It, Plot It
Since each data point is a snapshot, one of the first things organizations do with the KPI data they collect is plot it over time. The resulting time series can answer at least a couple of questions, such as, “Are there any detectable patterns?” and “Is this metric going in the right direction?”
Often the answer to first question (even if it’s a “yes”) is not very useful from a business standpoint. That’s because even if you can detect, for example, a day/night pattern in a business metric, there’s usually not much that you can do to change that pattern – the length of a day is stubbornly resistant to human influence. Since the only metrics you can improve are the ones you’re able to change, detecting a pattern like this is useless for providing actionable insights.
Unless of course you’re able to relocate to Mars, in which case enjoy your extra 40 minutes.
The answer to the second question is found by looking at the trend line, the general slope of the plot over a long period of time. Trend lines are important and informative, but they also have their limits. Perhaps the biggest limit is the fact that they are inherently long-term indications of data over time. By design, trend lines smooth out variations in the data, like a moving average.
A Trend Line Is Not the End of the Line
When those variations are the normal variability in the data, that smoothing is welcome. When those variations are a bend in the curve, the start of a new “normal,” that smoothing will hide an important turning point. Used indiscriminately, trend lines can actually prevent you from extracting insights from your data, and as you can imagine, hidden insights lead to missed opportunities and lost money.
That lost money can take the form of missed revenue because you had insufficient inventory of a product that suddenly surged in popularity. Another example is an uptick in users leaving your platform due to a brand-devastating “babby”-grade meme gaining circulation on social media.
Both of those examples require quick action, but you can’t act on something you don’t know is occurring, and you don’t know something like this is occurring unless you can detect it in the data. Trend lines are useless for detecting business incidents like these because it can take a lot of unusual data points to accumulate before the trend line significantly changes.
Meanwhile, you’re hemorrhaging revenue and users. To stop the bleeding, you need a rapid response, and that requires quick detection of these deviations in the data (also called anomalies) long before they shift the overall trend. This is why savvy companies are moving to real-time anomaly detection.
Recommended Upgrade: Anomaly Detection
But what is anomaly detection? From our experience at Anodot, a real time business analytics company and vendor of anomaly detection systems, anomaly detection accomplishes three main things:
- Creates a model of “normal” values for a given business metric, including any patterns that may be present in the data;
- Uses the model to predict what the next data point should be; and
- Determines if that next data point matches what the model predicted, or is an anomaly.
This three-step process naturally lends itself to automation via software, which is a good thing because in some organizations, the number of monitored business metrics can number in the thousands or even millions. Another reason why anomaly detection wins over trend lines: An anomaly doesn’t have to be drastic in order to be costly. Detecting subtle anomalies requires sophisticated AI-powered software that catches these events, yet doesn’t flood you with false-positives.
Your data can only reveal as much as your analysis tools allow. Basic tools like trend lines can mask significant events until it’s too late. Anomaly detection can produce much faster results, and do so at the speed of today’s business environment.
Rebecca Herson is Vice President, Marketing for Anodot. She can be reached at rebecca@anodot.com.